The introduction of the first weather satellites in 1960 provided meteorologists with a new tool to observe weather globally, including regular monitoring of the development of tropical cyclones like hurricanes and typhoons for the first time in remote locations of the world’s oceans. Over the following decades, improvements in the satellites as well as the remote sensing technologies employed by them have allowed scientists to develop new techniques to characterize these storms remotely.
From 1969 to 1984, American meteorologist Vernon Dvorak (National Environmental Satellite, Data, and Information Service – NOAA) developed a technique to estimate the strength of tropical cyclones based on the storm’s morphology as observed by weather satellites (Dvorak 1975, 1984). In the decades to follow, the Dvorak Technique has been improved to provide ever more accurate assessments by incorporating more satellite data but, it has never approached the accuracy of characterizations afforded by Hurricane Hunter aircraft. Even in its latest incarnation, the automated Advanced Dvorak Technique can only estimate the central pressure of tropical cyclones to an accuracy of ±15 hPa (hectopascals – the equivalent of millibars frequently used in the US). Still more refined and less subjective techniques under development which incorporate a wider range of satellite remote sensing data, like SATCON being developed by Christopher Velden and Derrick Herndon (Cooperative Institute for Meteorological Satellite Studies, University of Wisconsin – Madison), promise to improve the estimation of tropical cyclone central pressures to the ±6 hPa level leaving more room for improvement (Herndon 2018; Velden 2020).
A New Approach – The Carnot Engine Model
A new approach to determine the strength of tropical cyclones was formulated in 1994 by Kerry Emanuel (MIT and a member of the TWA Science Advisory Board) and Paul Joss (MIT and a now-retired cofounder of TWA). In 1988, Emanuel estimated the maximum theoretical strength of tropical cyclones by treating these storms as a thermodynamic system known to physicists as a Carnot Engine (Emanuel 1988). Working for Visidyne, Inc. (the corporate antecedent of TWA), Emanuel and Joss found that the strength of a tropical cyclone could be determined using this model in combination with overhead remote sensing data from satellites (Emanuel 1994).
Tropical cyclone (TC) structure is presented in Figure 1. The primary wind field is rotational and is the cause of damage to life and property. The rotation is generated by the Coriolis force acting on the lower air mass as it moves toward the low-pressure area at the TC center. The secondary wind field (inward toward the eye at the surface, up the eyewall, toward the outer edge of the storm at the tropopause, then subsiding at the outer edge) controls the storm energetics. This secondary wind field, whose effects are observable from overhead, is the subject of the Carnot Engine model.
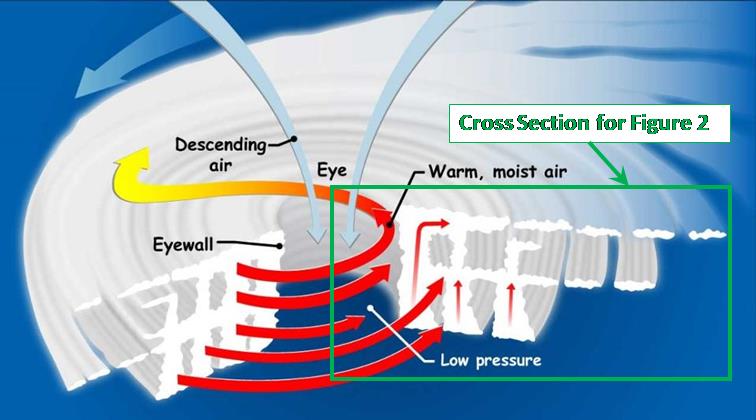
Professor Emanuel’s description of the model is presented in Figure 2. In Leg 1 air spirals inward from the outer regions along the sea surface boundary layer about 1 kilometer thick. Contact with the surface ensures that the flow is approximately isothermal and large quantities of heat are added by evaporation from the warm water. In Leg 2 air ascends the eyewall and flows out to large distances. This leg is nearly adiabatic if the working fluid includes the combination of dry air, water vapor, and condensed water. In Leg 3 air descends in the lower stratosphere, while losing heat by radiation to space. This leg is nearly isothermal owing to the isothermal structure of the lower stratosphere. In Leg 4 air descends back to the sea surface. Because the ambient atmosphere has a temperature lapse rate that is nearly moist adiabatic, the net entropy loss in this leg is very small.
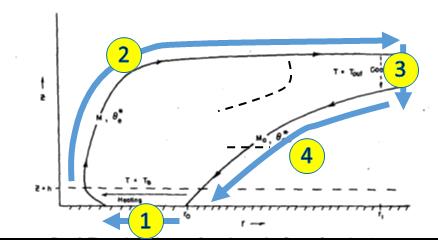
The key inputs to the Carnot Engine model are the altitude and temperature of the eyewall cloud tops, as well as the sea surface temperature. If these can be measured with sufficient accuracy, the cyclone central pressure can be determined much more accurately than with current satellite remote sensing methods. With the central pressure known, several semi-empirical models are available to relate the storm surface pressure to the maximum sustained winds (Ariffin 2009; Knaff 2007; Holland 2007).
Examples of sensitivity of the Carnot Engine model predictions to the input parameter values are presented in Figures 3 and 4. Figure 3 shows sensitivity of central pressure ratio (i.e. the central pressure divided by the ambient atmospheric pressure of 1013 hPa) to cloud top altitude for a sea surface temperature of 300 K and six values of cloud top temperature. Figure 4 shows sensitivity to cloud top temperature for the same sea surface temperature and four values of cloud top altitude.
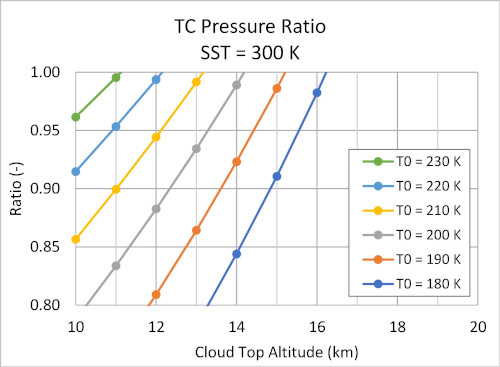
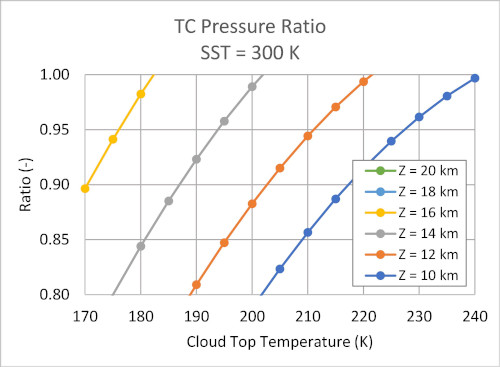
The estimated uncertainty of the TC intensity (as a function of the measurement uncertainty in the eyewall cloud top temperature and altitude) calculated using the Carnot engine model is shown in the simplified plot shown below in Figure 5. The uncertainties associated with well-established techniques, like the Modified Dvorak Technique with central pressure uncertainties in excess of 15 hPa, typically fall towards the outer bounds of Region III of this figure. Newer techniques currently under development, like SATCON with central pressure uncertainties of about 6 hPa (Herndon & Velden 2018), fall in the inner part of Region II and would provide a substantial improvement. Employing the Carnot engine model using cloud top temperature and altitude measurements with uncertainties of better than ±1 K and ±100 m, respectively, are well within reach of the current state-of-the-art satellite-based remote sensing techniques and would result in central pressure uncertainties of about ±3.5 hPa. Falling inside Region I of Figure 5, this approach promises to provide excellent estimates of TC intensity.
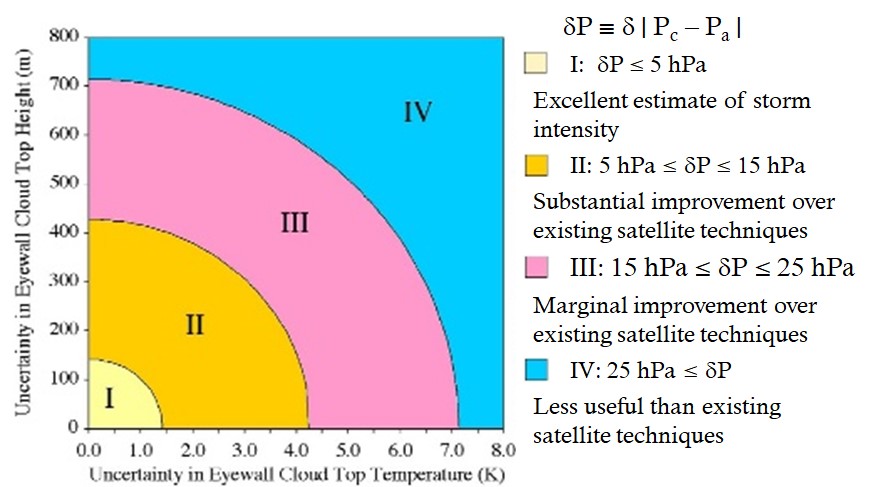
Validation of the Carnot Engine Model Using Satellite Data
In order to validate the Carnot engine model for intense TCs, Luo (2008) analyzed near-simultaneous cloud top altitude and temperature measurements of TCs derived from instruments on board the EOS A-Train satellite constellation. For the cloud top altitudes, data from CloudSat’s CPR (Cloud Profile Radar) with an altitude accuracy of 480 meters were used while the 11 μm band data from MODIS (Moderate Resolution Imaging Spectroradiometer) on Aqua obtained within about 60 seconds of CloudSat were used to determine cloud top temperatures. Sea surface temperatures for this analysis were derived from Reynolds-weekly-average 1° latitude/longitude data to estimate the sea surface temperature along each segment of the CloudSat and Aqua orbit under each TC. The measurement uncertainties of this work places it towards the inner part of Region III in the uncertainty plot of Figure 5. They examined 150 overpasses from the July 2006 to January 2007 to find a total of nine which included measurements over or near the eye of TCs. A sample of CloudSat and MODIS data of Hurricane Ileana in the East Pacific obtained on August 23, 2006 is shown below in Figure 6.
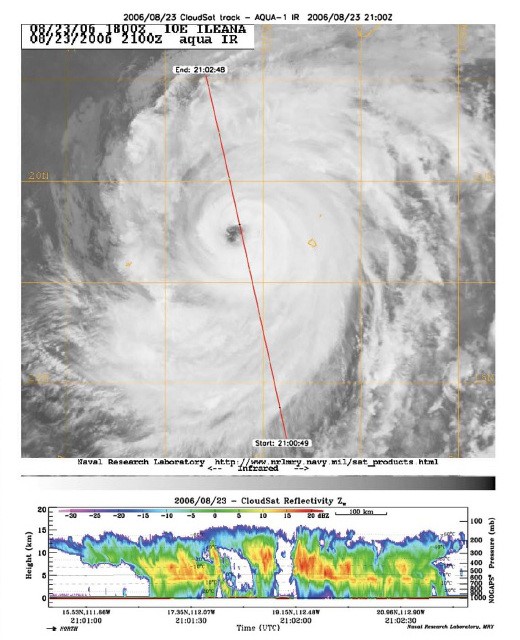
For their analysis, Luo (2008) employed two different methods for estimating the change in moist static energy, Δh, in the TC eye: one which relies on the slope of the cloud top altitudes moving away from the eye and another which uses the sea surface temperatures while assuming a relative humidity of 80%. As shown in Figure 7 below, their preliminary analysis showed promising results with their calculated peak surface winds agreeing well with the best track maximum sustained winds especially for the three Category 3 and 4 TCs examined. While Luo (2008) stated “the analysis that was described in this letter is far from being mature enough to be converted to an operational algorithm for estimating storm intensity”, the results were important because it represented a unique and first-of-a-kind test of a theory of TC intensity which is physics-based as opposed to earlier empirically-based remote sensing methods which rely on the correlation of similarities in multiple observable storm characteristics to estimate TC strength.
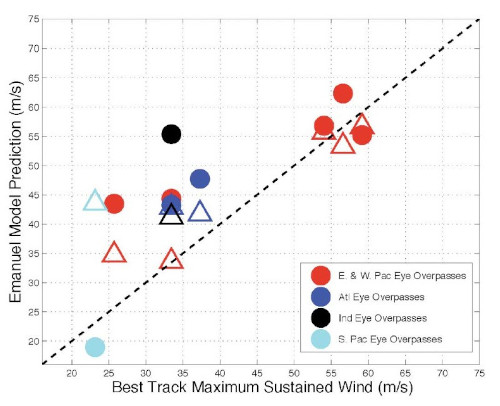
The Carnot engine model for TCs was also used in two other investigations employing alternate remote sensing methods for determining key model inputs. Sieron (2013) used a convective-permitting Weather Research and Forecasting V3.3.1 simulation of Hurricane Katrina during a six-day period in August 2005 to assess the proposed use of cloud-top pressure data products of the type generated from MODIS and GOES observations as an alternate means of deriving Δh with promising results.
Vergados (2014) used dual-frequency GPS radio occultation observations made by two different low Earth orbit (LEO) satellites (i.e. Challenging Minisatellite Payload and COSMIC RO) to measure the vertical temperature profiles through the eyes of 27 TCs observed between 2004 and 2010. These data provide accurate measurements of not only the difference between the sea surface and cloud top temperatures at the TC eyewall, but also more accurate constraints on the value of Δh. Once again, this approach resulted in TC intensity measurements with useful accuracy and precision across the range of TC strength from “tropical storm” to Category 5 (with RMS errors in calculated surface wind speeds of 4.0 and 4.5 m/s, depending on the assumptions made about the wind speed gradient), as shown in Figure 8 below. While the remote sensing methods employed in Sieron (2013) and Vergados (2014) to derive needed inputs for the Carnot engine model differ from Luo (2008) as well as that which we propose to use, they nonetheless provide additional evidence for the potential accuracy of the Carnot engine model compared to other approaches.
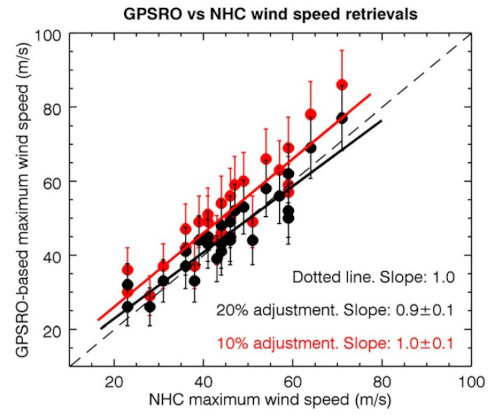
The Hurricane Hunter Satellites
Despite the promising potential of using the Carnot Engine model to characterize the strength of hurricanes, typhoons, and other tropical cyclones using overhead remote sensing data, reliable sources of the required data are simply not available today. Orbiting lidars and radars capable of making measurements of cloud heights to the required accuracy are typically confined to making measurements at nadir along the satellites’ ground track. With ground tracks typically spaced ~2,300 kilometers apart in the tropics during successive orbits, the chances that such a satellite will make cloud altitude measurements through the eye any given TC is only on the order of ~2%. Likewise, the randomly scattered data points from the growing constellations of satellites using the GPS radio occultation method are also unlikely to probe the eye of any given TC during the course of a day. Other satellite-based techniques to measure wind speeds of a TC over the ocean, like microwave scatterometry or radar, become increasingly inaccurate at windspeeds above 100 mph (45 m/s) because of the frothy state of the sea surface under those conditions.
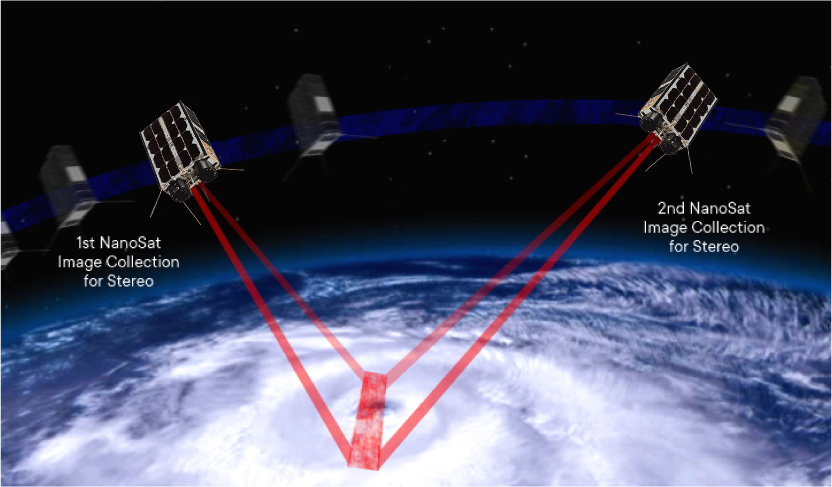
TWA is developing a constellation of 6U CubeSats, called the Hurricane Hunter Satellites, specifically designed to gather the overhead remote sensing data required for a practical implementation of the Carnot Engine model. Operating in pairs, each Hurricane Hunter Satellite will be equipped with a camera array capable of acquiring overlapping, 2,000-kilometer wide swaths of images with a pixel scale of about 100 meters. Analysis of these stereographic image swaths will provide cloud height measurements with a typical uncertainty of 100 meters. With a constellation of five pairs of satellites in separate orbital planes, any given TC can be observed about once every 2½ hours during daylight hours (for more details, see “The Hurricane Hunter Satellites”). Combined with cloud top temperature data provided by the latest generation of GEO weather satellites and sea surface temperatures available from a range of sources, the Carnot Engine model can be used to characterize TCs around the globe on a regular basis. This information, added to the unique 3D wind measurement capability possible with the Hurricane Hunter Satellites’ stereographic imaging technique, promises huge advances in our ability to accurately forecast the future path and strength of hurricanes and other tropical cyclone worldwide.
Additional Reading
Drew LePage, “The Hurricane Hunter Satellites”, Drew Ex Machina, May 15, 2022 [Post]
Drew LePage, “Turning Up the Stereo”, TWA Blog, March 3, 2022 [Post]
References
Ariffin, Munirah, and Subramaniam Moten, “Relationship Between Maximum Sustained Wind Speed and Central Pressure of Tropical Cyclones”, Research Publication No. 1/2009, Malaysian Meteorological Department, 2009
Dvorak, Vernon F., “Tropical Cyclone Intensity Analysis and Forecasting from Satellite Imagery”, Monthly Weather Review, Vol. 103, No. 5, pp. 420-430, May 1975 [Paper]
Dvorak, Vernon F., “Tropical Cyclone Intensity Analysis Using Satellite Data”, NOAA Technical Report NESDID 11, September 1984 [Report]
Emanuel, K. A., “The Maximum Intensity of Hurricanes”, Journal of Atmospheric Science, Vol. 45, No. 7, pp. 1143-1155, April 1988 [Paper]
Emanuel, K. A., “A Satellite-Based Stereographic Technique for Estimating the Intensity of Tropical Cyclones”, White Paper Prepared for Visidyne, Inc., 1994
Herndon, D. and C. Velden, “An Update on the CIMSS SATellite CONsensus (SATCON) Tropical Cyclone Intensity Algorithm”, 33rd AMS Conference on Hurricanes and Tropical Meteorology (Ponte Vedra, FL; April 16-20, 2018), 2018 [Abstract]
Holland, Greg, “A Revised Hurricane Pressure-Wind Model”, Monthly Weather Review, Vol. 136, No. 9, pp. 3432-3445, September 2008 [Paper]
Knaff, John A., and Raymond M. Zehr, “Reexamination of Tropical Cyclone Wind-Pressure Relationships”, Weather and Forecasting, Vol. 22, No. 1, pp 71-88, February 2007 [Paper]
Luo, Z., G. L., Stephens, K. A. Emanuel, D. G. Vane, N. Tourville, and J. M. Haynes, “On the Use of CloudSat and MODIS Data for Estimating Hurricane Intensity”, IEEE Geoscience and Remote Sensing Letters, Vol. 5, No. 1, pp. 13-16, January 2008 [Paper]
Sieron, Scott B., Fuqing Zhang and Kerry A. Emanuel, “Feasibility of tropical cyclone intensity estimation using satellite-borne radiometer measurements: An observing system simulation experiment”, Geophysical Research Letters, Vol. 40., pp. 5332-5336, doi:10.1002/grl.50973, 2013 [Paper]
Velden, Christopher S. and Derrick Herndon, “A Consensus Approach for Estimating Tropical Cyclone Intensity from Meteorological Satellites: SATCON”, Weather and Forecasting, Vol. 35, No. 4, pp. 1645-1662, August 2020 [Paper]
Vergados, Panagiotis, Zhengzhao Johnny Luo, Kerry Emanuel and Anthony J. Mannucci, “Observational test of hurricane intensity estimations using GPS radio occultations”, Journal of Geophysical Research: Atmospheres, Vol. 119, pp. 1936-1948, doi:10.1002/2013JD020934, 2014